Conducting a Network Meta-analysis (NMA)
Project #1: Establishing a new standard for comparing data across clinical trials
For this project, we developed new methodological approaches to adjust networks of valid but disconnected data to create larger, more connected, and more accurate data models. Evidence bases often differ among clinical trials, producing information networks that are difficult or impossible to compare directly. In order to provide reliable estimates of relative efficacy among all relevant biological psoriasis treatments in a study population, researchers from Analysis Group developed an innovative and influential way to establish cross-trial similarity.
Conventional network meta-analyses (NMAs) that synthesize all available evidence from randomized clinical trials are able to deliver only partial comparisons using incomplete datasets. However, the reliability of this evidence depends on data similarity across trials. We noted that there was substantial variation in the response rates of reference arms (control groups of patients) across key psoriasis trials, which can be a source of significant bias in a traditional NMA. To overcome this potential bias, our team introduced a new methodology to adjust for variations in reference-arm response within a network meta-analysis.
The reference-arm-adjusted models fit the clinical trial data significantly better than unadjusted models, and led to a reliable estimation of relative efficacy among all relevant biological treatments in the study population. As a result, the reference-arm-adjusted model is now the standard model expected in psoriasis submissions to the National Institute of Health and Care Excellence (NICE) in the UK, which is the economic decision maker for the UK’s National Health Service. In the years since we developed this methodology, top-tier clinical journals have published a number of reference-arm-adjusted NMAs across multiple disease areas.
As the figure below shows, our models helped clinicians compare Humira to other therapies, even when clinical trials had not compared those drugs head to head. The standard we developed allows clinicians and patients to draw comparisons among drugs and make better-informed decisions when selecting treatments.
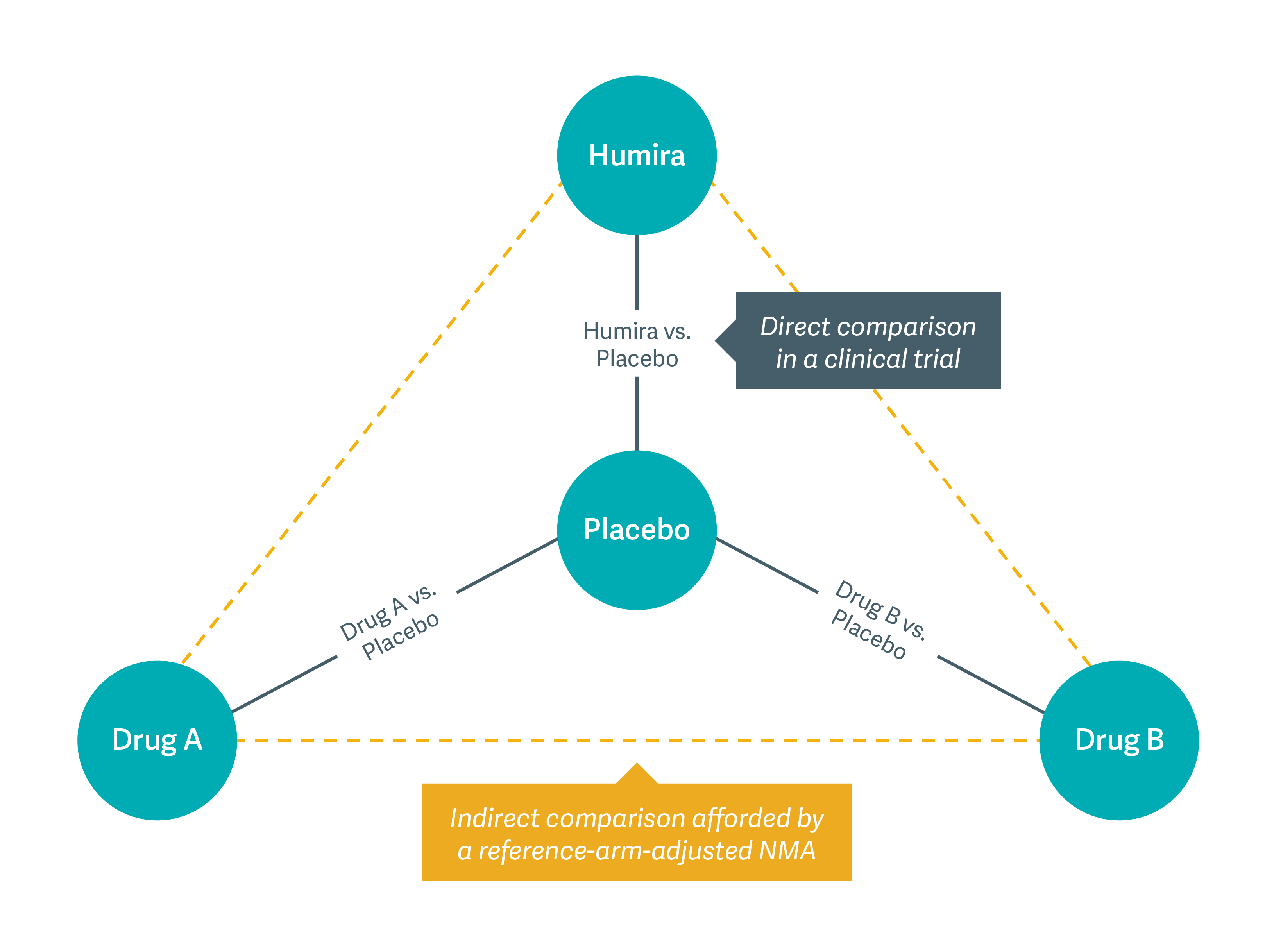
Project Team Member Profiles
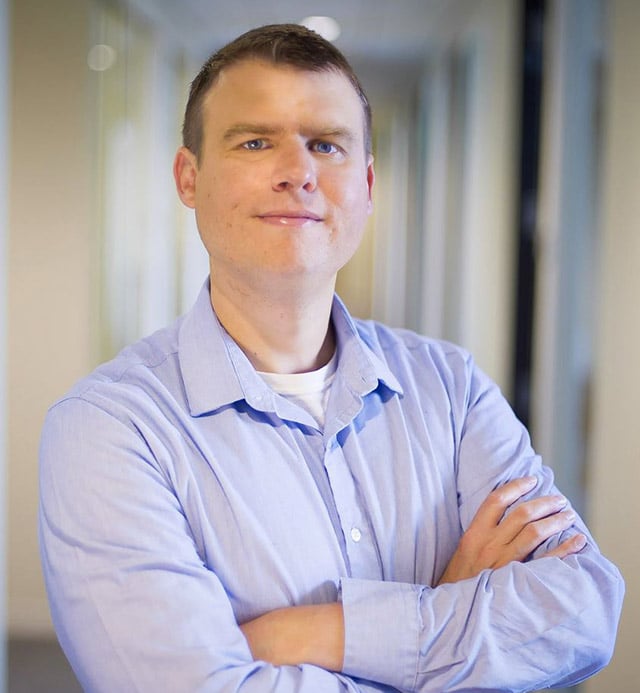
Keith Betts
Associate (now Managing Principal), Los AngelesI worked on this reference-arm adjustment project when I was an associate. I had completed my Ph.D. degree in biostatistics, and had a special interest in comparative-effectiveness research. I had previously discussed the idea of extending traditional meta-regression in the network meta-analysis setting with my colleagues on the Humira team. It wasn’t until we were trying to wrap our heads around surprising results in a traditional network meta-analysis of psoriasis treatments that we conceptualized the oversized impact of differences in reference-arm response. We shared our observations with the client, and partnered in this collaborative research project. The collaboration resulted in this successful innovation, which has since become the standard practice within psoriasis treatment and across network meta-analyses.
This model has been updated many times over the years. For each new psoriasis treatment that comes to market, Analysis Group again runs the model and assesses the comparative effectiveness across the current psoriasis landscape. Over time, a number of talented team members who began working on this project as junior staff members have transitioned into more senior project management roles.
This project required a vital combination of strong technical skills and collaboration. It provided an outlet for creativity and outside-the-box thinking. The project was a huge success for both the drug developer and Analysis Group, and it provided team members with excellent training and experience.